Event on AI-driven Decision Intelligence 04 July 2024
Our event on AI-driven Decision Intelligence in at ZHAW in Winterthur, Switzerland on June 26, 2024 was a major success as it provided a fruitful platform for discussions to likeminded professionals, and exchange with industry leaders. I am happy that seven referees presented major success stories in real-world applications of AI, aiming for sustainability and efficiency goals. The talk by Swissport offered an in-depth view on the challenges to optimize the shift rostering of over 2000 employees at the Zurich airport, each with a lot of special constraints. Axpo, the largest energy provider in Switzerland, showed how they schedule maintenance operations of hydropower dams. The interactive workshops and panel discussions were an interesting addition to our conference with over 65 participants. And last but not least, the Swiss-style apéro offered a perfect opportunity to network. Let me thank again the sponsors Ateleris, Gurobi, and KPMG for their generosity.
The Fourth International Workshop on Dynamic Scheduling Problems 10 November 2023
The Fourth International Workshop on Dynamic Scheduling Problems IWDSP 2023 was a complete success. Scientists from all over the world came together at the ZHAW to exchange their latest research results on dynamic scheduling.
Of particular note was the plenary lecture on computational complexity in scheduling under stochastic influences with scenarios by Prof. Leen Stougie (former group leader at Centrum Wiskunde & Informatica, the Dutch national research institute for mathematics and computer science, and professor of computer science at the Free University of Amsterdam, the Netherlands).
While the conference took place in the historical physics building in reminiscence of Prof. Albert Einstein, we can now publish our proceedings of the talks digitally. Furthermore, we are pleased that the renowned Journal of Scheduling will publish a special issue on our workshop.
The workshop was organized in Winterthur by Dr. Helmut Sedding, Operations Research and Operations Management, Institute for Data Analysis and Process Design, ZHAW School of Engineering under the scientific direction of Prof. Stanisław Gawiejnowicz, Algorithmics, Faculty of Mathematics and Computer Science, Adam Mickiewicz University of Poznań, Poland and Prof. Gur Mosheiov, Operations Research and Operations Management, Charles I. Rosen Chair in Business Administration, Hebrew University of Jerusalem, Israel.
We would also like to acknowledge the support of the scientific and local committees and the Swiss National Science Foundation with the SNSF Scientific Exchanges project no. 211197.
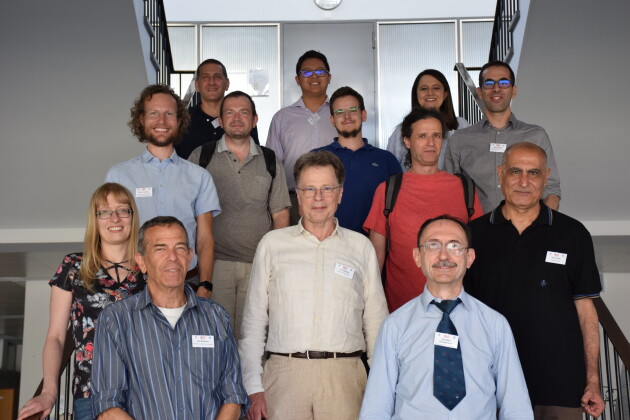
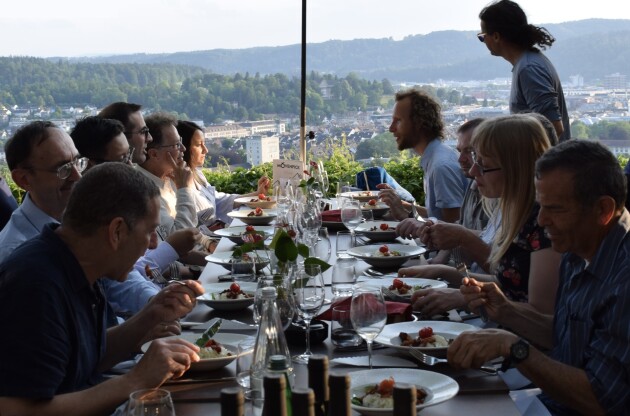
Reload on QML code change 28 August 2023
Developing a Qt app with QML has the advantage that there exists an interpreter for QML code. This enables to reload code without restarting the Qt application unless there is a change in compiled code. We present a novel and simple implementation of such a reloading functionality. This can noticeably speed up development of QML applications.
There are several existing approaches described and found online. Most of them use a separate class to implement the functionality. This is extensible, but requires to create and reference extra files. An alternative way is to use the official tool qmlpreview.
Here, we strive for short code that is integrated in a simple way by adding it to the main function. We assume that
- all QML code has ‘qml’ and ‘js’ extensions,
- is added to the project’s qrc file,
- and is stored relative to the project root.
We conditionally include
#ifdef QT_DEBUG
#include <QFileSystemWatcher>
#include <QDirIterator>
#endif
and add a few lines to the main function; located after declaring QQmlApplicationEngine engine and setting its import path, and located before entering Qt’s application loop:
#ifdef QT_DEBUG
engine.addImportPath(QFileInfo(__FILE__).absolutePath());
QFileSystemWatcher watcher;
for(QDirIterator it(":", {"*.qml", "*.js"}, QDir::Files, QDirIterator::Subdirectories); it.hasNext();)
watcher.addPath(QFileInfo(__FILE__).absolutePath() + it.next().mid(1));
QObject::connect(&watcher, &QFileSystemWatcher::fileChanged, [&](){
engine.clearComponentCache();
engine.load(QFileInfo(__FILE__).absolutePath() + "/main.qml");
});
#endif
First, we ensure that the current file path is the first and foremost import path for the QML engine. This ensures that changed files are loaded from disk, not from the compiled qrc file. Then, the qrc file is scanned for the ‘qml’ and ‘js’ files. These are added to Qt’s file system watcher to listen for updates. On update, the QML engine cache is cleared and the main.qml file is loaded again.
We note that when reloading several files, the code is reloaded several times. This redundancy is avoided by collecting the changes and reloading only once. We attain this by adding a single shot QTimer.
#include <QTimer>
…
#ifdef QT_DEBUG
engine.addImportPath(QFileInfo(__FILE__).absolutePath());
QFileSystemWatcher watcher;
for(QDirIterator it(":", {"*.qml", "*.js"}, QDir::Files, QDirIterator::Subdirectories); it.hasNext();)
watcher.addPath(QFileInfo(__FILE__).absolutePath() + it.next().mid(1));
QTimer asyncifyWatcher;
asyncifyWatcher.setSingleShot(true);
QObject::connect(&watcher, &QFileSystemWatcher::fileChanged, [&](){
asyncifyWatcher.start(10);
});
QObject::connect(&asyncifyWatcher, &QTimer::timeout, [&](){
engine.clearComponentCache();
engine.load(QFileInfo(__FILE__).absolutePath() + "/main.qml");
});
#endif